How Applied Materials and fab partners are harnessing machine learning
The equipment giant’s Computational Process Control strategy takes a pragmatic approach to Industry 4.0 and is likely to influence EDA tools for incoming nodes.
Applied Materials, the largest supplier of semiconductor production equipment, is working with fab owners to develop ramp and yield strategies based on machine learning. It sees the overall effort as building a new factory paradigm, Computational Process Control (CPC).
At Semicon China, Applied corporate vice president Kirk Hasserjian described the CPC concept and cited examples of its use so far.
Four important points could be drawn from Hasserjian’s presentation:
- Increasing process complexity now makes the use of some kind of AI-based analysis necessary, particularly as the industry moves toward 5nm. As the number of process steps grows and the tools used in fabs become more complex, so the amount of data that needs to be addressed is “skyrocketing”.
- At the same time, the move to completely AI-based machine learning is still some way off. Considerable human expertise in processes, materials and equipment is needed and will be for some time yet to understand and tune these new strategies.
- Delivering CPC requires even closer collaboration across the semiconductor supply chain than is already taking place. Big data adds a new dimension on top of the existing yield learning curve and methodology.
- The results of CPC will feed back up into the EDA design flow – much as has been seen in, for example, lithography – to make designs sufficiently robust and fab-ready for incoming nodes.
Data drivers for Computational Process Control
From Applied’s perspective, CPC is enabling it to reach an overarching goal it has had for many years. The company develops and delivers the tools to make the chips. CPC then helps it and its customer “increase good die out as fast as possible”.
It is an evolution beyond the “statistical process control” introduced into fabs during the 1980s and 1990s, and the “advanced process control” added early in this century to provide more detailed feed-forward and feedback loops following the move to 300mm wafers.
This evolution is largely driven by the explosion in ‘Big Data’ as tools necessarily become more complex, face more potential sources of error or yield loss, are required to undertake more process steps (Figure 1), and therefore undergo a vast increase in the number of sensors needed to monitor their operation.
Hasserjian explained that this data can be analyzed within two types of model: supervised and unsupervised. The latter is arguably closer to what many people think of as pure machine learning.
“In unsupervised models, you have ‘unlabeled data’ and you are essentially looking for groupings and trends, identifying anything that is anomalous,” Hasserjian said. “There’s quite a bit of data coming out of our processes and tools that you can use that modeling for.”
However, he argued that the supervised model is equally, if not more important right now. These have understanding within them. They do a better job of separating the signal from the noise.
“There are two other pieces that are critical when you are dealing with new technologies and sophisticated new tools,” Hasserjian said.
“There’s the equipment understanding and expertise – knowing what components are used and what materials are used, how the tools are cleaned and conditioned, and so on.
“The other piece is the process side. These tools were designed to help customers on certain processes. Understanding those processes is also key. The equipment suppliers have some of that understanding but the semiconductor manufacturers have a tremendous amount into how those process steps are integrated overall.”
For the supervised model, that knowledge needs to be inserted into the CPC analysis to get the best results.
“In supervised modeling, you do have a closer understanding of the data. That is considered ‘labeled data’, and you can use that labeled data to train your algorithms and models to really identify problem areas: what’s normal and what’s not normal.”
Figure 2 breaks down the supervised and unsupervised opportunities as they stand: supervised components are shown in the yellow boxes on the left; unsupervised ones in the grey boxes on the right.
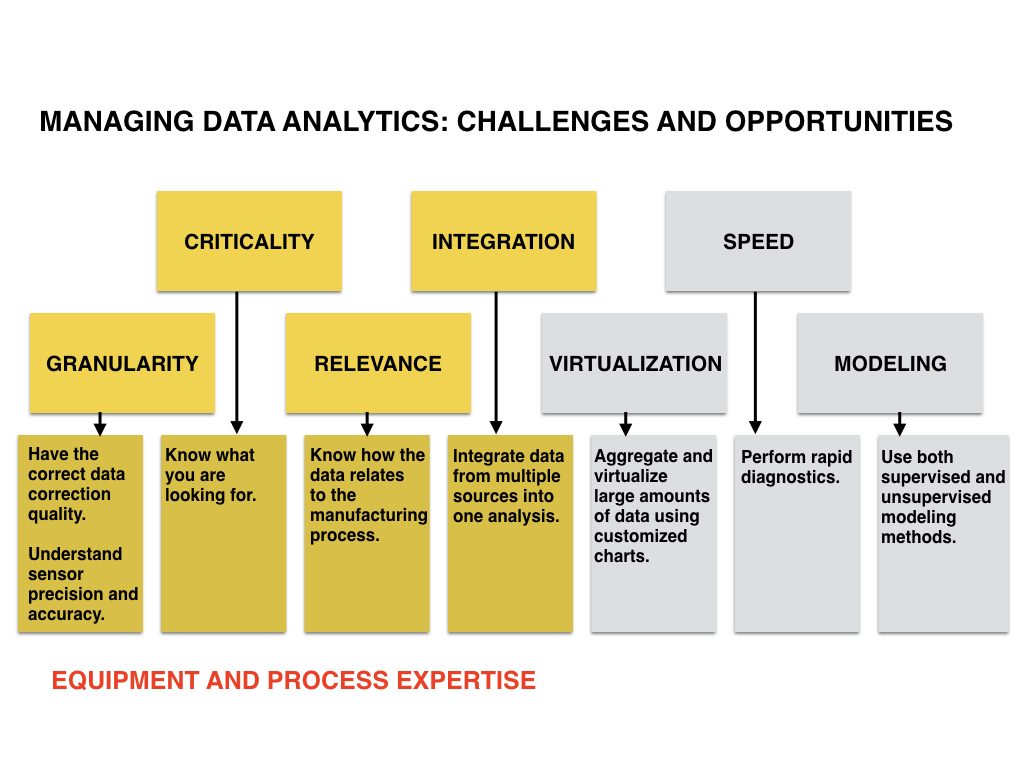
Figure 2. Where human knowledge feeds Computational Process Control (Applied Materials – click to enlarge)
“Going forward, we think that the industry will move to a semi-supervised approach where you have both supervised and supervised models in the overall factory analysis. That is really the direction we see things going when we talk about machine learning,” said Hasserjian.
As of today, this will provide major efficiencies but not the kind of wholesale automation in analytics that has been suggested.
“Completing that total digital twin [of the factory] is not achievable today, but the different pieces are coming together,” Hasserjian said. “At the tool level, there is already a lot of activity and it will have a huge impact when you can simulate the entire factory like that to optimize output. But it is not there now, though one day it will be.”
Computational Process Control demands greater collaboration
Given the ongoing need to insert a lot of human experience into the CPC process, it is self-evident that that success will require the broadest participation.
“Another side of this is the need to connect Computational Process Control to a comprehensive knowledge network,” said Hasserjian. “That will help ensure that you have the best knowledge and understanding of how the process runs and how the tools perform. It will ensure that the best-known practices are readily available in the factory. That requires very close collaboration between suppliers – both equipment and materials – and semiconductor manufacturers.”
The high level overview of CPC in Figure 3 gives one idea of how such a network would comprise various contributors to the supply chain.
“So, how would this all come together on a real-world basis? You would have your tools and metrology and they need to be hooked to a server that looks at the entire fleet of tools you have, all done over secure networks,” Hasserjian explained. “Then, that is also connected to your knowledge network, and that network may be made up of experts who can look at the data remotely and help you understand what kind of issues can be seen.”
The role of EDA tools in this kind of learning infrastructure is probably going to start as an extension of that already played in the design-for-manufacturing space.
“I see [EDA] as a very fertile field to move this concept into,” said Hasserjian. “Already some of those tools have a very strong influence on the integrated manufacturing process flow, in etching, in lithography, and in patterning. Translating the kind of algorithms from CPC into those tools could certainly improve the robustness of the designs arriving at the fab.”
Computational Process Control in practice
CPC is more than just a theory. Applied Materials has already started to put the concept to work, and Hasserjian described some use cases at Semicon China.
In one, a CPC approach delivered a close match using a virtual metrology model for the thickness uniformity of a PVD film when compared with an actual deposition, based on a heat map.
“This can be used for many purposes. For example, one is tool surveillance. If you are working against a good virtual model and there are any shifts, the hundreds of sensors on the actual tool can pick them up in real-time, and before you might have a yield excursion or a downtime event,” said Hasserjian.
In another, CPC with human input was used to focus on priority sensor signals to create a multivariate health index for etch chambers. Here, individual signals did not provide a sense of why process shift was being observed during qualification, but by pulling on the knowledge network approach to know which signals to combine, the system could detect anomalies much earlier.
“This saves significant time in terms of the number of wafers you have to run before you can start production,” said Hasserjian.
In each case, he noted that the system worked where it could draw upon “a lot of subject matter expertise”.
Being more battle-hardened as a concept, CPC offers a practical take on how machine learning, AI and other automation techniques can be adopted in the broader context of what is now called Industry 4.0.
Semiconductor manufacturing is about as complicated a factory task as you can imagine, so you can see the ongoing need for human input to both identify more valuable algorithmic opportunities and tune them to optimize performance. Other industries with less complex production processes may move toward the digital twin more quickly.
But in terms of multi-billion-dollar fab investments, pragmatism isn’t a bad watchword.