Yield is money – and other truths of diagnosis-driven yield analysis
Diagnosis-driven yield analysis identifies the cause of systematic yield loss to speed the ramp-to-volume on new processes and improve yield on mature ones.
Finding the root causes of yield loss is becoming yet more challenging as the number and complexity of design-sensitive defects increases. Yield issues present several bottom-line concerns:
- Missed market windows. Spend months chasing down root causes and your product my reach its market too late.
- Uncertain circuit reliability. Yield excursions can imply reliability problems. An unstable yield means discarding wafers that do not meet quality requirements.
- Lost profit. Digging out that last extra 1-2% of mature yield can significantly boost the bottom line, particularly for high-volume ICs.
To help locate defects that lead to yield loss, engineers have typically used scan diagnosis. Once a handful of defective devices is found that represent a yield problem, the team employs diagnosis software to identify the most likely defect locations. This location-finding process is refined further through electrical and physical fault isolation prior to construction analysis.
But what if you could improve on this approach so that rather than just using diagnosis for defect localization, it could be extended to determine the underlying root causes and best physical failure analysis (PFA) candidates in a batch such as a wafer or lot of failing devices?
This extended methodology is known as diagnosis-driven yield analysis (DDYA). It uses production test results, volume scan diagnosis, and statistical analysis to identify causes of yield loss prior to failure analysis. It represents an advance on existing techniques in many ways, including improved PFA results, reduced PFA time, much faster location of root cause(s) much faster, and even the discovery of previously hidden yield limiters.
Mentor, A Siemens Business, has developed a DDYA approach (Figure 1) that uses its Tessent Diagnosis and Tessent YieldInsight products. These can reduce the time needed to detect a route cause by 75-90%, and identify systematic yield limiters that would otherwise never be found.
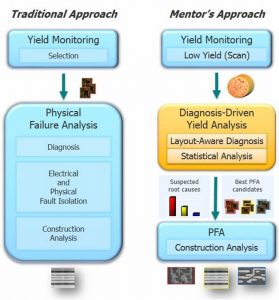
Figure 1. Traditional yield strategy compared with one using diagnosis-driven yield analysis (Mentor – click to enlarge)
Using diagnosis for yield analysis
Two components must be in place to make diagnosis-driven yield analysis happen:
- The diagnosis needs to produce the type of data that is meaningful for yield learning.
- The analysis of diagnosis results must consider inherent ambiguities within those results.
Getting meaningful data
This process involves leveraging layout information like cell type, via type, layer, and critical area. Compared to a diagnosis based simply on a logic-only design description (netlist), a layout-aware diagnosis improves the diagnosis resolution and provides additional defect classifications. Moreover, because as much as 50% of defects in today’s advanced manufacturing processes can be internal to the cells, a transistor-level (cell-aware) diagnosis using fault models derived from analog simulation is very beneficial.
For identification of scan chain defects and chain-functional compound defects (10-30% of logic failures), Mentor’s Tessent Diagnosis DDYA tool includes advanced scan chain diagnosis. To identify delay defects and timing errors, it also includes an at-speed diagnosis capability. You also need a lot of data to effectively leverage diagnosis for yield analysis, so the process must be run on production test patterns in the presence of scan test compression, and with minimum impact on test time. Tessent Diagnosis directly diagnoses test results based on compression-mode Tessent TestKompress patterns, as well as Tessent FastScan patterns.
Considering ambiguities
This means separating the valuable information from the noise. Mentor’s Tessent tools use two main techniques:
- Zonal analysis. This analyzes the distribution of a defect signature to help spot patterns that point to systematic issues.
- Root cause deconvolution (RCD). This is a statistical enhancement technology that increases the PFA success rate and dramatically reduces the PFA cycle time from months to days (Figure 2). Where layout-aware diagnosis points to a segment, RCD can isolate a particular root cause in that segment.
To help resolve systematic defects that are design-process related, DDYA can be combined with design profiling for a DFM-aware yield analysis. In DFM-aware yield analysis, the goal is to identify the DFM rules that best describe the actual design-process induced systematic defects.
There have been some challenges to realizing this approach. For example, when you find a defect location that correlates with a DFM violation, how do you know that the violation is the actual cause of the defect? And what if a defect is caused by a design feature that isn’t modeled by the existing DFM rules?
To deal with such issues, Mentor correlates layout-aware diagnosis data with Critical Feature Analysis (CFA) results from Calibre YieldAnalyzer. If a suspect defect is partially or fully in the same layer and location as a DFM rule violation, this suspect is said to correlate with that DFM rule (Figure 3).
Diagnosis-driven yield analysis case study
At the 2012 SEMICON China (CSTIC), Mentor and Freescale Semiconductor (now part of NXP Semiconductors) described how they enabled mature yield improvement with diagnosis-driven yield analysis. Freescale had a high-volume design in final production and wanted to improve the yield to achieve greater cost savings. The initial analysis with traditional yield methods showed that the current mature yield loss was caused by random (baseline) defects. Using these methods, any further analysis would be very time consuming and expensive.
For a DDYA experiment, 1,300 failure files were collected out of several lots of failing devices. (each file represented a scan test failing die). Mentor and Freescale collected these failing cycles and performed volume diagnosis. The diagnosis results were then loaded into Tessent YieldInsight where wafer maps showed a random failure distribution. They selected the remaining logic-only-failure dice (around 700) and analyzed all signatures across all the zonal types. When the analysis was complete, the possible yield signatures were shown in the Tessent tool with different colors (Figure 4-1). In this case, the ‘Suspect Region: Count of Die’ was flagged as a higher possible yield signature in zonal type Y. It implied that defect locations were sensitive to a specific layout region (Figure 4-2).
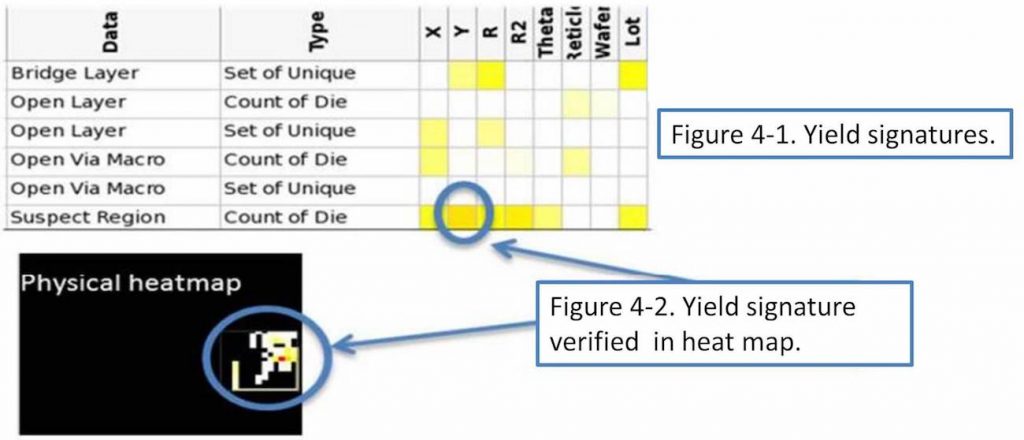
Figure 4. Yield signatures shown in the Tessent Diagnosis console and confirmed in a heat map of the layout (Mentor – click to enlarge)
There were 30 dice associated with this suspect region. The majority of the failures were due to open layers (including vias) ranging from M1 to M5 based on an open layer signature. The wafer map of these 30 dice showed that the top Y region was highly correlated with the hotspot. This information helped guide which die to choose for PFA. The failing information (location along with failing type) was given to the foundry for PFA and there were three PFA hits out of five die total. The foundry made a process correction based on the three PFA results, and as a result, the mature yield was improved by 1.7% – three times the original goal. The analysis and process correction was completed in just a few weeks, and significantly faster than what could have been achieved without the Tessent tools.
Summary
An effective yield analysis flow can be realized by combining highly accurate volume scan diagnosis in Tessent Diagnosis with visualization and statistical analysis in Tessent YieldInsight. Applying yield analysis based on volume scan diagnosis results that incorporate design layout and failure data, rather than relying on manufacturing process data alone, can reduce the cycle time to finding the root cause of yield loss by 75-90%. As designs increase in complexity and process technology advances, the statistical noise reduction of RCD assists in reducing diagnosis noise and cell-aware diagnosis detects transistor-level defects. The diagnosis-driven yield analysis approach can be supplemented with DFM-aware yield analysis to separate design and process related yield limiters.
About the author
Matthew Knowles is the Diagnosis and Yield Analysis product marketing manager at Mentor, a Siemens Business.