The path to full functional monitoring
The growing demands upon electronics system design are helping to chart a path towards platforms that will not only deliver optimal silicon but also allow far more detailed insights into how entire systems perform throughout their lifecycles.
That was one of the main takeaway from a recent strategic keynote by Joe Sawicki, Executive Vice President, IC EDA at Siemens Digital Industries Software, to users of the company’s software, in which he looked at where trends are taking the industry today and in the near future.
Sawicki’s main argument was that design strategies are undergoing major and necessary change in three main areas (also shown in Figure 1).
- SoC creation: now moving towards techniques that allow more early-stage architectural exploration – pre-RTL if possible – to strike the right balance between the increasing processing muscle demanded by AI and machine learning and the need to achieve this at the lowest viable power consumption. Siemens is already well positioned here with its high-level synthesis offerings.
- SoC verification and validation: with the validation side becoming ever more important as the critical question facing these processes become not just, “Does it meet spec?” but increasingly, “Can it actually run the software?”
- Digitization of the environment: the necessary step that allows that validation to be carried out thoroughly in a digital twin of likely real-world operating conditions. The digital twin provides the technique that supports a move towards what Sawicki called true “functional monitoring”.
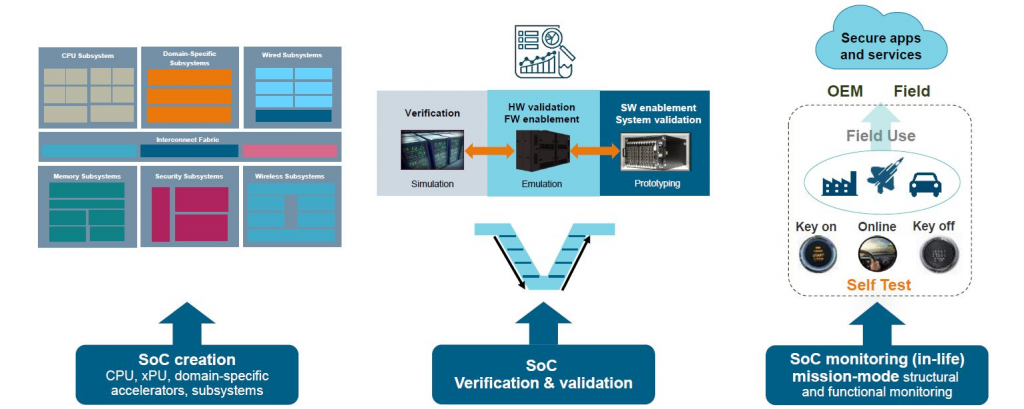
Figure 1. Enabling the digital twin transforms SoC design and deployment (Siemens – click to enlarge)
Beyond verification to validation
Sawicki offered a new spin on Mark Andreessen’s famous observation that software is eating the world. “There is a somewhat less poetic, more corollary to that, and it is that software performance is going to define semiconductor success.”
Beyond cache coherency, memory interconnect, safety, security, and power, success will first and foremost be determined by the “actual performance executing on software”, particularly as machine learning and AI play a greater role in systems generally. A large part of achieving that will come down to detailed, useful and usable metrics, and being able to run software against hardware earlier in the design process. Sawicki described what this kind of environment should look like in detail (also summarized in Figure 2):
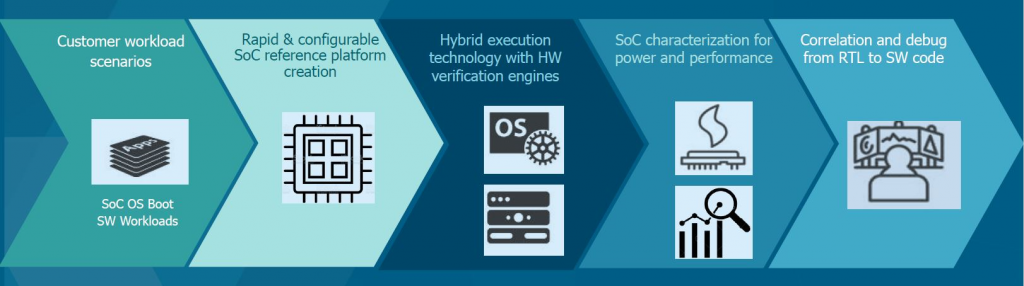
Figure 2. What is requried to solve the billions-of-cycles software workload characterization problem (Siemens – click to enlarge)
“First off, we meet need to be able to handle and enable the presence of customer workloads. Whether those be industry-standard benchmarks that need to be met, specific applications that are germane to your implementation, or things like graphics testing. They then need to be able to be fed into an easily configurable system that can…bring together virtual models for a CPU, a gate-level implementation for perhaps an accelerator, and perhaps RTL for a memory subsystem management tool; and then [allow you to] swap [things] in and out depending upon the maturity of those parts of design in a quick manner.”
Sawicki added that there need to be engines underneath this type of configuration that are “running things like CPU models, running simulations on a standard type of netlist and bringing in hardware-assisted verification tools like emulation and prototyping to get to the amount of cycles through the design necessary to be able to validate full software stacks”.
All this needs to be supplemented by analytical tools that allow the user to focus on and fully understand what impact applications are having on power and performance. The environment should therefore allow for the concurrent management of software and hardware debug, so that they can efficiently identify the issues that cause a design to miss performance specifications.
Overall, digitization is the key enabler in terms of delivering the levels of integration, visibility, granularity and efficiency that this strategy needs.
Beyond validation to digitization
Sawicki cited PAVE 360, Siemens automotive-aimed digital twin environment, as an example of how the digitization process can be broadened out from the SoC validation environment to one that locates it within a greater system-of-systems, and apply stimuli that approximate those that will be encountered in the real world:
“Whether these [conditions] include other drivers, pedestrians, traffic, lighting, traffic control systems, the data can then be fed into the virtual sensors for the device, fed into the electronic system, and then used to control models of both the powertrain and the chassis, feeding that back then to virtualized environment.
“So, we can actually run a complete virtualized environment with the semiconductors operating well before that anyone has ever even thought about pushing the tape-out button to go to silicon.”
He said that this same approach is now being applied to 5G to model the behavior of electronics in circumstances akin to those at the edge of a network or in a cell tower, integrating elements such as channel models, degradations in transmission and interfaces to the cloud. “We can model the entire stack again and look at how these 5G applications will perform,” Sawicki said.
After also embracing some of the latest innovations in test around areas such as scan and compression alongside all these extra features and other digitization elements, Sawicki said that Siemens is moving toward a platform that will allow for the design-in of IP to perform functional monitoring, the real-time analysis of a design in operation (Figure 3).
“What’s going to be possible with this type of technology?” Sawicki asked. “Well, it ranges from basics like doing bus monitoring to do something like detecting a stuck pixel that may be affecting the overall ability to process imaging data as it’s coming into the system,” he explained.
“Then, it could be something like looking at thread switching or out-of-bounds execution where a Trojan or some other type of attack is coming onto the chip that can be detected by watching what’s happening with the [CPU] address bus.
“Or more sophisticated tools could be able to bring out that data, feed it up into the cloud and run machine learning-based detection to look at more subtle types of issues that may occur, whether those be from a reliability or from a data-integrity perspective.”
There is then a good deal more to this than simply taking the lemons and making lemonade out of the costs of seemingly ever-increasing design complexity. Rather, in Sawicki’s view, “We think there’s an opportunity to take the complexity that’s going to occur because of what we’re to implement and turning that into an advantage for us all in terms of enabling this digital twin, giving better insights and better designs over time to become more successful in the market.”